A Proven AI PRD Template by Miqdad Jaffer (Product Lead @ OpenAI)
Build and scale AI-powered products successfully. A Product Requirements Document template with an AI PRD case study for Shopify Auto Write.
Hey, Paweł here. Welcome to the free special edition of The Product Compass Newsletter.
With 103,731 Product Managers from companies like Meta, Amazon, Google, and Apple, this newsletter aims to be #1 source for learning and growth as a PM.
Expect actionable tips, templates, resources, and insights.
No fluff. Every week.
Consider subscribing and upgrading your account for the full experience:
In 2025, the AI market is estimated to be around $638 billion. It’s expected to reach $1,811 billion in 2030 with a CAGR (compound annual growth rate) of 36.6%.
It’s an excellent opportunity for AI Product Managers. In the U.S., Senior AI PMs already earn $259K per year on average.
But not every AI implementation is successful.
Given the hype around AI, many implement AI without a clear, justified business case. To make things worse, AI-specific considerations are often overlooked.
Today’s guest,
, works as a Product Lead at OpenAI, helping OpenAI’s partners succeed. He previously worked on AI as the Director of Product at Shopify.For the first time, Miqdad shares his AI PRD (Product Requirements Document) template, tested in the trenches, that:
Addresses common problems with AI implementations.
Provides critical guidance to help you make better decisions.
Can be applied to most AI products and features (especially LLM-powered).
It will help you implement and scale AI-powered solutions successfully.
We discuss:
CHAPTER I: How to Work With the AI PRD
CHAPTER II: AI PRD Template: Key Sections & Guidance
CHAPTER III: AI PRD Template to Download (Google Docs)
CHAPTER IV: AI PRD Case Study for Shopify Auto Write
CHAPTER V: Conclusions
Before we proceed, I’d like to recommend the AI Product Management Certification. It’s a six-week cohort taught by instructors like Miqdad Jaffer.
I (Paweł) participated in the cohort in Spring 2024. The next session starts on April 26, 2025.
I was able to secure a $250 $500 discount for our community if you use this link to sign up:
This post was not sponsored.
CHAPTER I: How to Work With the AI PRD
Before we dive into the AI PRD template, let’s briefly discuss how to use it.
1. AI PRD as a Powerful Alignment Tool
In my experience (Miqdad), an AI PRD is the core of the AI product development lifecycle. In many organizations I work with, it is the primary alignment tool used during Product Review Meetings (PRMs) to achieve cross-functional consensus around product strategy, design, implementation, and go-to-market activities.
The AI PRD sits at the intersection of strategy, planning, detailed product development, and effective go-to-market execution.
It covers three areas:
Strategic Context: Defines why your company should build this product or feature, clearly identifying strategic alignment, market growth, and competitive advantage.
Product & Technical Excellence: Clearly outlines how your product creates value, detailing key features and capabilities, technical specifications, and AI-specific considerations that are often overlooked.
Go-to-Market: Guides how you effectively communicate, launch, measure, and continuously improve your product to maximize customer and business value.
2. AI PRD as a Living Document
The AI PRD is a living document. In our AI Product Management course, we discuss the concept of "continuous PRM mode" - a mindset in which Product Managers constantly operate in a state of testing assumptions and gathering insights, as visualized by Paweł:
Every interaction, whether a Slack conversation, hallway discussion, formal meeting, or customer feedback session, becomes an opportunity to test assumptions about user value, usability, business viability, and feasibility.
These insights should continuously update your AI PRD, ensuring it remains accurate and relevant.
CHAPTER II: AI PRD Template: Key Sections & Guidance
Here, I outline each PRD component with a clear understanding of its purpose, recommended tools, examples, and common traps.
The specific sections of your AI PRD should be customized to your context, including the company's strategy, market position, product scope, and user base.
In the next chapter, we discuss how this framework was applied to Shopify Auto Write.
1. Executive Summary
Purpose: Briefly summarize the initiative and success criteria for those who won’t read the entire document.
Example:
“This product requirement document describes building an AI assistant integrated with the existing customer support chat.
It answers to an identified market opportunity (25% CAGR), is aligned with our company strategy, and leverages our unique competitive edge (proprietary data) with the pilot phase planned for the end of Q2 2025.
Success criteria:
An 80% satisfaction (CSAT) rating.
50% of user requests are solved automatically.
Generate accurate responses to the 25 most frequently asked FAQs.”
2. Market Opportunity
Purpose: Clarify if the opportunity is strategically compelling, timely, and positioned to deliver meaningful value.
Key Questions:
Is this the right market growth stage (e.g., emerging, maturing, declining)?
What is the market’s current growth rate (e.g., CAGR), and what data supports this?
What’s the potential for this opportunity in the future? Can it create enough business value?
Recommended Tools:
Industry Lifecycle Model (related to market and business growth)
Technology Adoption Lifecycle (how users adopt innovative products or technologies)
Example of Good Response:
"Market X has shown a consistent growth rate of 25% CAGR due to recent regulatory changes and customer adoption increasing exponentially. Currently, it's at an early growth stage (10-15% market penetration), providing ample room for capturing substantial value. TAM projected at $5B by 2030."
Example of Bad Response:
"Our competitors are entering this market, so we should too."
Common Traps to Avoid:
Misinterpreting temporary trends as long-term market shifts.
Failing to validate growth assumptions.
Ignoring external macroeconomic or regulatory factors.
Advice: Hard data beats gut feelings. Quantify the opportunity with market reports or customer surveys.
3. Strategic Alignment
Purpose: Articulate how your AI product or feature aligns with your vision, strategy, and objectives.
Key Questions:
For an AI product:
Does this align with our company’s vision and long-term strategy?
How does this support our company objectives?
Does this play to our strengths and competencies?
For an AI feature:
Does this align with our company’s vision and long-term strategy?
Does this align with our product vision and strategy?
How does this support our team objectives?
Recommended Tools:
Lean Canvas (unlike Business Model Canvas, it defines “unfair advantage”)
Product Strategy Canvas and Startup Canvas (add vision, tradeoffs, and capabilities)
Product Mix Strategy (for managing and optimizing product portfolio)
Example of Good Response:
"Our AI-driven risk assessment product aligns with our company’s focus on the finance sector. It directly supports our mid-term objective of increasing adoption of 20% mid-market bank adoption by automating regulatory compliance workflows."
Example of Bad Response:
"We’ll use AI because it’s innovative and trending."
Common Traps to Avoid:
Not tying the initiative to your company/product vision, strategy, and objectives.
Ignoring the company’s competencies and resources.
Advice: Not every problem is the right one for your team or organization.
4. Customer & User Needs
Purpose: Prioritize solving the highest-value user problems clearly and precisely.
Key Questions:
What are the key market segments and user personas?
What are their primary jobs-to-be-done and pain points?
Are there any constraints (e.g., geographic, language, regulatory)?
Which user problems, if solved, would generate the most frequent, severe, and widely experienced value?
Recommended Tools:
Opportunity Score by Dan Olsen (importance vs. satisfaction)
Jobs-to-be-Done Framework (more time-consuming if performed rigorously)
Example of Good Response:
"Users spend two hours daily manually reviewing data reports, resulting in frequent errors. 80% of users report this as their top pain (survey, n=200, extremely high importance, extremely low satisfaction)"
Example of Bad Response:
"Users have said our current solution is not user-friendly."
Common Traps to Avoid:
Choosing low-frequency or low-severity user pains.
Assuming user pain without direct evidence or validation.
Over-generalizing user needs without specifics.
Advice: Use interviews, surveys, or (for an existing product) analytics to understand and build empathy with the users rather than assuming their jobs and pain points.
5. Value Proposition & Messaging
Purpose: Communicate the unique value proposition clearly and compellingly.
Key Questions:
Which problems for each market segment do we address?
What are the key capabilities and features (high-level) that solve those problems?
What are the benefits and customer outcomes?
How is this different from what others offer?
How can we clearly and compellingly communicate our product’s unique value to resonate with each segment?
Recommended Tools:
Value Proposition Template (in their post, How to Design a Value Proposition,
and explain how they added focus and structure to the Value Proposition Canvas)Value Curve (to compare your value proposition with competitors’).
Example of Good Response:
"Our product reduces customer support workload by 40%, saving 10 hours weekly, clearly addressing frequent and painful user bottlenecks with AI assistants available 24/7."
Example of Bad Response:
"Our product is powerful and innovative."
Common Traps to Avoid:
Generic messaging without specific, measurable user outcomes.
Listing features and capabilities without benefits.
Listing benefits without explaining how they will be achieved.
Failing to align messaging with specific market segments.
Advice: Adjust messaging and format to each segment and channel (e.g., cost savings for budget users and short videos on Instagram).
6. Competitive Advantage
Purpose: Clarify your product’s defensibility and ability to sustain competitive advantage.
Key Questions:
What makes us think competitors can’t/won’t copy our strategy?
How defensible and durable is our advantage in the long-term?
Recommended Tools:
Competitive Advantage (MOATs)
Example of Good Response:
"The product leverages our proprietary dataset of over 10 M+ transactions and integrates seamlessly with our existing fraud detection system, giving us a 3-year competitive lead.
While other solutions rely on generic machine learning models, our domain-specific AI is trained on industry-specific workflows, making it even harder to replicate.”
Example of Bad Response:
"Our UI is more user-friendly than competitors."
Common Traps to Avoid:
Vague or unclear differentiation statements.
Relying on easily replicable advantages like superficial UI features.
Underestimating competitor agility or resources.
Choosing differentiation based solely on technology.
Neglecting reassessment of competitive advantage. Competitive edges erode.
Advice: Consider hard-to-replicate edges like data, partnerships, or integrations.
7. Product Scope and Use Cases
Purpose: Define the key capabilities and features with tasks our product (or a feature set) must perform exceptionally well.
Key Questions:
What are the key capabilities and features?
Can we link designs or prototypes for better alignment?
What are the desired customer outcomes?
What are the high-risk assumptions? How can we test them with minimal effort?
Recommended Tools:
Low-fidelity and high-fidelity user prototypes.
Market engagement experiments, usability testing, spikes, and experiments on production. A comprehensive overview: Testing Product Ideas.
Example of Good Response:
An AI assistant integrated with the existing customer support chat. It must generate accurate responses to the 25 most FAQs, achieving an 80% satisfaction (CSAT) rating.
Design: [Figma prototype]"
Example of Bad Response:
"The AI assistant will answer questions users might have."
Common Traps to Avoid:
Trying to include too much information (e.g., including all user stories or describing every corner case) - your PRD is a tool for building alignment, not explaining every detail.
Not specifying measurable customer outcomes.
Trying to address all user needs simultaneously rather than starting simple and iterating based on customer feedback.
Neglecting to test high-risk assumptions before implementation.
Advice: A picture is worth a thousand words. Show, don’t just tell.
8. Non-Functional Requirements
8.1 General Requirements
Purpose: Define the essential system attributes (e.g., performance, scalability, security) that ensure the product operates reliably under expected conditions.
Key Questions:
What technical, performance, scalability, security, and reliability attributes must our product achieve to deliver and sustain its intended value?
What are the peak load and user volume expectations?
Recommended Tools:
MoSCoW Method (Must, Should, Could, Won’t) for prioritization.
Performance benchmarking tools (e.g., JMeter, LoadRunner).
Security compliance checklists (e.g., OWASP Top 10, GDPR).
Example of Good Response:
"The AI assistant: delivers responses in <500ms for 95% of queries, scales to 50K users with 99.9% uptime, encrypts all user data per GDPR standards."
Example of Bad Response:
"The system should be quick and secure enough for users."
Common Traps to Avoid:
Overlooking scalability needs until late in development, leading to costly rework.
Writing vague requirements (e.g., "fast" or "reliable") without measurable targets.
Failing to balance trade-offs between attributes (e.g., performance vs. cost).
Advice: Define specific metrics early. Remember that “fast” isn’t a target.
8.2 AI-Specific Requirements (LLMs)
Purpose: Ensure the AI consistently delivers accurate, reliable, ethical, and user-aligned outputs.
Key Questions:
What are the key AI architectural choices?
What accuracy, reliability, and ethical standards must our AI meet (e.g., best practices, brand voice, and legal compliance guiderails)?
How will we measure these qualities?
How will we maintain them over time?
Recommended Tools:
AI evaluation metrics (e.g., Precision, Recall, F1 Score, BLEU for text).
Bias and fairness audits (e.g., Fairlearn toolkit).
Prompt engineering frameworks (e.g., chain-of-thought prompting).
Retrieval-augmented generation (RAG) for grounding outputs in verified data.
Additional Resources:
Scikit-learn: A widely-used Python library for machine learning that includes implementations of Precision, Recall, and F1 Score.
NLTK: The Natural Language Toolkit (NLTK) provides an implementation of BLEU (Bilingual Evaluation Understudy), commonly used to evaluate text generation
Fairlearn Toolkit: An open-source Python package from Microsoft for fairness assessment and mitigation in machine-learning models.
Example of Good Response:
"The AI must achieve ≥90% accuracy on a labeled test set of 10,000 queries, limit hallucination rates to <2% via RAG integration, and flag inappropriate outputs with 98% precision, validated monthly through human review."
Example of Bad Response:
"We’ll use GPT and expect good accuracy because it’s popular."
Common Traps to Avoid:
Using pre-trained models without validation or fine-tuning.
Neglecting ongoing monitoring for drift in accuracy or reliability.
Ignoring ethical risks (e.g., bias, inappropriate outputs) until user complaints arise.
Advice: Monitor drift. Without proper oversight, retraining, and adaptation, an AI system can become outdated and unreliable (e.g., due to changes in the relationship between input data and the expected outputs). Regular monitoring, validation, and feedback loops are essential.
9. Go-to-Market Approach
Purpose: Define how you rapidly demonstrate measurable value and grow user adoption.
Key Question:
What are the build and release phases (e.g., an MVP addressed to early adopters)?
What are the first market segment(s) we want to focus on?
How can we win those customers, rapidly demonstrate the value, and get evidence to accelerate further growth?
Recommended Tools:
Crossing the Chasm Framework (e.g., to target early adopters)
AARRR (Pirate) Metrics (e.g., to scale while tracking Acquisition, Activation, etc.)
Example of Good Response:
"We’ll launch an MVP targeting small e-commerce businesses in North America, offering 20% cost savings on customer support within 30 days. Success will be measured by a 50% activation rate and 10 case studies in 90 days, driving expansion to mid-sized companies.
We believe that the next step will be…"
Example of Bad Response:
"We’ll launch on Product Hunt and see what happens."
Common Traps to Avoid:
Targeting an overly broad or poorly defined initial segment(s), diluting focus.
Skipping measurable success metrics.
Confusing Early Customer Profiler (ECP) with Ideal Customer Profile (ICP).
Advice: Start small, measure the results, and learn fast rather than trying to make it perfect. At the same time, test responsibly (e.g., company reputation). Use early wins to fuel growth.
CHAPTER III: AI PRD Template to Download (Google Docs)
[Edited] As requested, we’re adding a Google Docs version of the PRD template.
CHAPTER IV: AI PRD Case Study for Shopify Auto Write
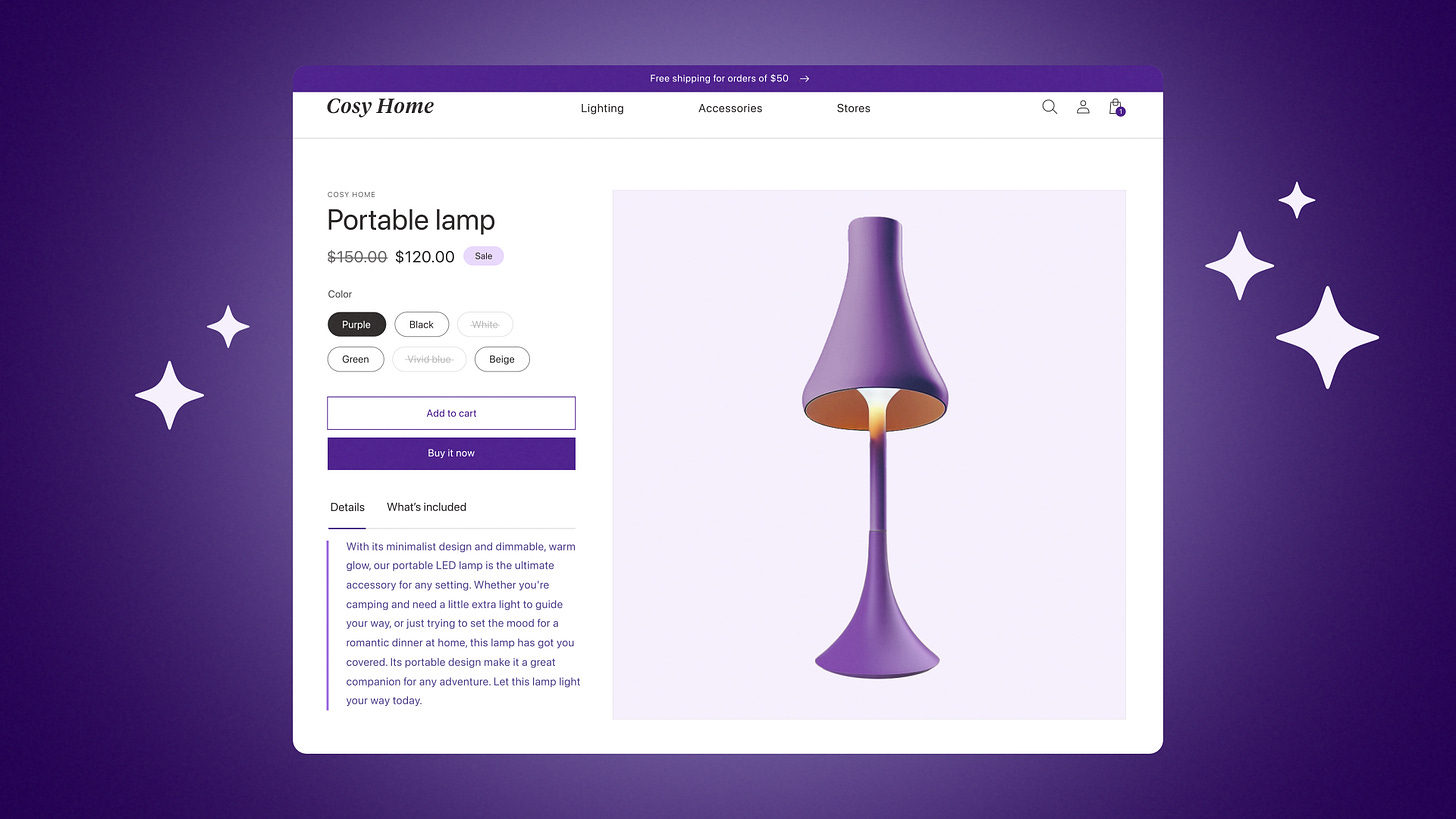
This section applies the AI PRD framework to a real-life AI-powered feature I (Miqdad) worked on in Shopify called "Auto Write." It is a product description automation tool leveraging Large Language Models (LLMs).
1. Executive Summary
Purpose: Briefly summarize the initiative and success criteria for those who won’t read the entire document.
Shopify’s Response (Good Example):
“This product requirement document outlines Shopify’s Auto Write, an AI-powered feature leveraging Large Language Models (LLMs) to automate product description generation for merchants.
It aligns with the growing e-commerce market opportunity (17% GMV growth to $55B in Q2 2023) and Shopify’s merchant-first strategy, capitalizing on our competitive edge in platform integration and proprietary data.
The launch is planned for Q3 2023. The pilot will target small and new merchants to enhance efficiency and SEO performance.”
2. Market Opportunity
Purpose: Clarify if the opportunity is strategically compelling, timely, and positioned to deliver meaningful value.
Key Questions:
Is this the right market growth stage (e.g., emerging, maturing, declining)?
What is the market’s current growth rate (e.g., CAGR), and what data supports this?
What’s the potential for this opportunity in the future? Can it create enough business value?
Shopify’s Response (Good Example):
In 2023, Shopify saw a growing need for merchant efficiency as e-commerce shifted rapidly online, with merchants demanding faster, high-quality content. Shopify’s Q2 2023 GMV grew 17% to $55B, reflecting this trend.
Shopify was strategically early on the curve of deploying practical LLM-based productivity tools at scale, capturing first-mover advantage in AI-generated e-commerce content.
Common Traps Avoided:
Avoided jumping on short-lived AI trends by focusing on a validated market need (productivity improvements).
Used GMV data to back the opportunity, not assumptions.
Factored in the broader shift to digital commerce, not just tech hype.
3. Strategic Alignment
Purpose: Articulate how your AI product or feature aligns with your vision, strategy, and objectives.
Key Questions for an AI Product:
Does this align with our company’s vision and long-term strategy?
How does this support our company objectives?
Does this play to our strengths and competencies?
Shopify’s Response (Good Example):
Auto Write was aligned with the company’s vision of making commerce simpler for merchants.
It supported Shopify’s focus on merchant efficiency, SEO optimization, conversion improvement, and reducing friction in the merchant onboarding journey.
It was aligned with Shopify’s strength in merchant-focused solutions and leveraged its deep e-commerce expertise.
Common Traps Avoided:
Avoided chasing AI for its own sake, linking Auto-Write to their merchant-first strategy.
Used their e-commerce platform strengths, not untested areas, to deploy Auto Write.
4. Customer & User Needs
Purpose: Prioritize solving the highest-value user problems clearly and precisely.
Key Questions:
What are the key market segments and user personas?
What are their primary jobs-to-be-done and pain points?
Are there any constraints (e.g., geographic, language, regulatory)?
Which user problems, if solved, would generate the most frequent, severe, and widely experienced value?
Shopify’s Response (Good Example):
High frequency: Shopify identified a frequent pain - merchants struggle with manually writing product descriptions, a tedious daily task.
High severity: This slowed merchants down severely, hurting SEO and conversion rates.
Wide magnitude: Almost every merchant faced this issue frequently, leading to meaningful widespread value.
Common Traps Avoided:
Shopify avoided focusing on niche, low-frequency use cases that wouldn't impact a broad merchant base.
5. Value Proposition & Messaging
Purpose: Communicate the unique value proposition clearly and compellingly.
Key Questions:
Which problems for each market segment do we address?
What are the key capabilities and features (high-level) that solve those problems?
What are the benefits and customer outcomes?
How is this different from what others offer?
How can we clearly and compellingly communicate our product’s unique value to resonate with each segment?
Shopify’s Response (Good Example):
Shopify Auto Write targets (Who) new and small merchants, (Problem) addressing their struggle with slow, manual product-description writing. Its (Features) AI-powered generation feature creates SEO-optimized descriptions instantly. This (Benefits) cuts writing time, improves conversions, and boosts SEO, helping merchants launch faster. Unlike (Differentiation) generic tools, it’s built into Shopify’s platform for seamless use.
Common Traps Avoided:
Shopify skipped vague buzzwords.
Instead, it defined clear benefits (time saved, conversions up) tied to merchant needs and explained how those benefits would be achieved rather than simply listing features.
Explained what’s different about Auto Write.
6. Competitive Advantage
Purpose: Clarify your product’s defensibility and ability to sustain competitive advantage.
Key Questions:
What makes us think competitors can’t/won’t copy our strategy?
How defensible and durable is our advantage in creating long-term?
Shopify’s Response (Good Example):
“Shopify Auto Write’s defensibility stems from its deep integration into the Shopify platform and access to proprietary merchant data, making it tough for standalone AI tools to compete. Rivals face adoption hurdles without Shopify’s ecosystem and workflow fit.”
Common Trap Avoided:
Shopify avoided weak, easily copied advantages, anchoring Auto Write in platform integration and data.
7. Product Scope and Use Cases
Purpose: Define the key capabilities and features with tasks our product (or a feature set) must perform exceptionally well.
Key Questions:
What are the key capabilities and features?
Can we link designs or prototypes for better alignment?
What are the desired customer outcomes?
What are the high-risk assumptions? How can we test them with minimal effort?
Shopify’s Response (Good Example):
“Key features include (tied to customer needs and value proposition defined previously):
AI-generated product descriptions
Human-in-the-loop pattern for editing AI-generated descriptions
AI support for both product description generation and editing
Multiple editing entry points (...)
Design: [Shopify Auto Write prototype]
The desired outcomes are:
User Adoption Rate: Target 15% WoW usage within 180 days.
Merchant Satisfaction: 80% of feedback submitted is positive.
Reduction in merchant churn: Reduce the time for stores to be published from the draft state.
SEO Impact: Monitor lift in organic traffic for those leveraging the feature.
High-risk assumptions were tested through usability testing and technical spikes before the implementation.”
Common Traps Avoided:
Shopify initially fell into the trap of trying to address all user needs, but it corrected its course quickly.
Auto Write was launched in 2023 with core functionality (e.g., standard tones/styles).
Shopify refined based on merchant feedback.
8. Non-Functional Requirements
8.1 General Requirements
Purpose: Define the essential system attributes (e.g., performance, scalability, security) that ensure the product operates reliably under expected conditions.
Key Questions:
What technical, performance, scalability, security, and reliability attributes must our product achieve to deliver and sustain its intended value?
What are the peak load and user volume expectations?
Shopify’s Response (Good Example):
Scale to 15% of merchants at peak load.
Perform load test to understand and confirm specific limitations (e.g., delivers descriptions in [X]ms for [Y]% of queries).
Provide a disabled state if/when there is an API outage.
Common Traps Avoided:
Shopify addressed technical, latency, scalability, and compliance requirements, avoiding vague targets.
It did it early, avoiding treating non-functional requirements as afterthoughts.
8.2 AI-specific Requirements
Purpose: Ensure the AI consistently delivers accurate, reliable, ethical, and user-aligned outputs.
Key Questions:
What are the key AI architectural choices?
What accuracy, reliability, and ethical standards must our AI meet (e.g., best practices, brand voice, and legal compliance guiderails)?
How will we measure these qualities?
How will we maintain them over time?
Shopify’s Response (Good Example):
Choose OpenAI GPT-3 (Davinci-003) with clear guardrails on outputs.
Stream model’s output for lower latency (display responses in real time).
Limit time (and debounce) between regeneration (to prevent excessive requests).
Provide guidance around the use of generated content.
Moderate generated content for iOS to align with Apple's App Store requirements.
Implement robust feedback loops for continuous model refinement and human-in-the-loop quality assurance measured by quarterly review cycles.
Common Traps Avoided:
Shopify avoided blindly trusting LLM outputs without ongoing validation.
9. Go-to-Market Approach
Purpose: Define how you rapidly demonstrate measurable value and grow user adoption.
Key Question:
Are there build and release phases (e.g., an MVP addressed to early adopters)?
What are the first market segment(s) we want to focus on?
How can we win those customers, rapidly demonstrate the value, and get evidence to accelerate further growth?
Shopify’s Response (Good Example):
Released Auto Write in 2023 as a pilot with minimal features within Shopify Magic.
Focused on early-adopter merchants needing content efficiency.
Targeted 15% merchant adoption within 180 days - realistic targets for conversion and efficiency improvements confirmed early through A/B testing.
Demonstrated value with faster content creation and conversion gains and used success stories to grow adoption.
Common Traps Avoided:
Shopify set clear metrics (15% adoption) and tested via A/B, avoiding vague or unrealistic goals.
Kept the scope tight with core features, iterating based on feedback instead of overbuilding.
Insights from Shopify Auto Write Case Study
Shopify identified a compelling market opportunity and tightly aligned the Auto Write product with the company’s vision and strategy.
They explicitly focused on solving high-frequency, high-severity merchant pain points (time-consuming product description creation).
They avoided common AI pitfalls by:
Testing their assumptions (in particular, usability).
Defining precise non-functional requirements and guardrails on AI outputs.
Staying lean (the pilot approach) rather than over-engineering the solution.
Designing feedback loops (both quantitative and qualitative "vibe checks") to inform continuous improvement.
CHAPTER V: Conclusions
This primer provided a comprehensive, structured approach to crafting effective AI Product Requirements Documents (AI PRDs).
It introduced the AI PRD as the core of the AI product development lifecycle. It connects strategic context, product excellence, and go-to-market, all anchored by a single, universal spine: value creation.
Each AI PRD section is designed to help you design an AI product or feature that solves critical user problems, aligns with your strategic strengths, and delivers measurable business impact in a competitive, scalable way.
Remember that the AI PRD isn't a static artifact. It’s a living, continuously updated alignment tool driven by constant testing and feedback loops.
By adopting the structured PRD framework outlined in this post, you can confidently navigate strategic, product, and technical complexities.
By anchoring each decision to clearly articulated value creation, you'll ensure clarity and cross-functional alignment, ultimately delivering sustained and measurable value to users and your business.
Let’s Keep in Touch
Where can you find Miqdad?
AI PM Cohort (Next: April 26, 2025; $500 discount)
Product Faculty’s AI Newsletter:
Thanks For Reading The Product Compass Newsletter
It’s fantastic to learn and grow together!
Here are our best AI PM posts you might have recently missed:
Consider subscribing and upgrading your account, if you haven’t already, for the full experience:
Have a great rest of the week,
Paweł
It was great collaborating with you on this Paweł, thanks for having me on the Product Compass newsletter!
Such a valuable resource! AI is transforming product development, and having a structured PRD template makes the process so much more efficient. Thanks for sharing!